Bayesian Learning Model Predictive Control for Process-aware Source Seeking
Classical source seeking algorithms aim to make the robot reach the source location eventually. We proposes a process-aware source seeking approach which finds an informative trajectory to reach the source location. A multi-objective optimization problem is formulated based on rewards for both the search process and the terminal condition. Due to the unknown source location, solutions are found through Bayesian learning model predictive control (BLMPC). The consistency of the Bayesian estimator, as well as the convergence of the proposed algorithm are proved. The performance of the algorithm is evaluated through simulation results. The process-aware source seeking algorithm demonstrates improvements over other classical source seeking algorithms.
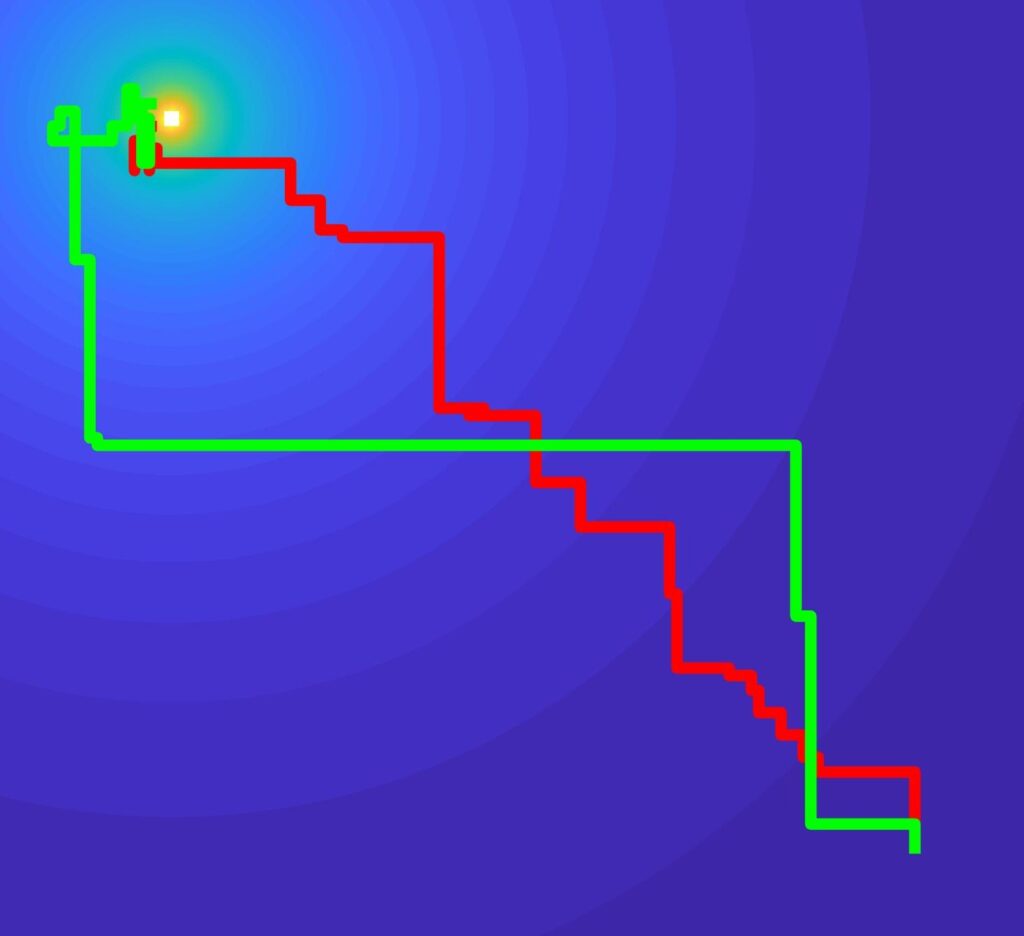
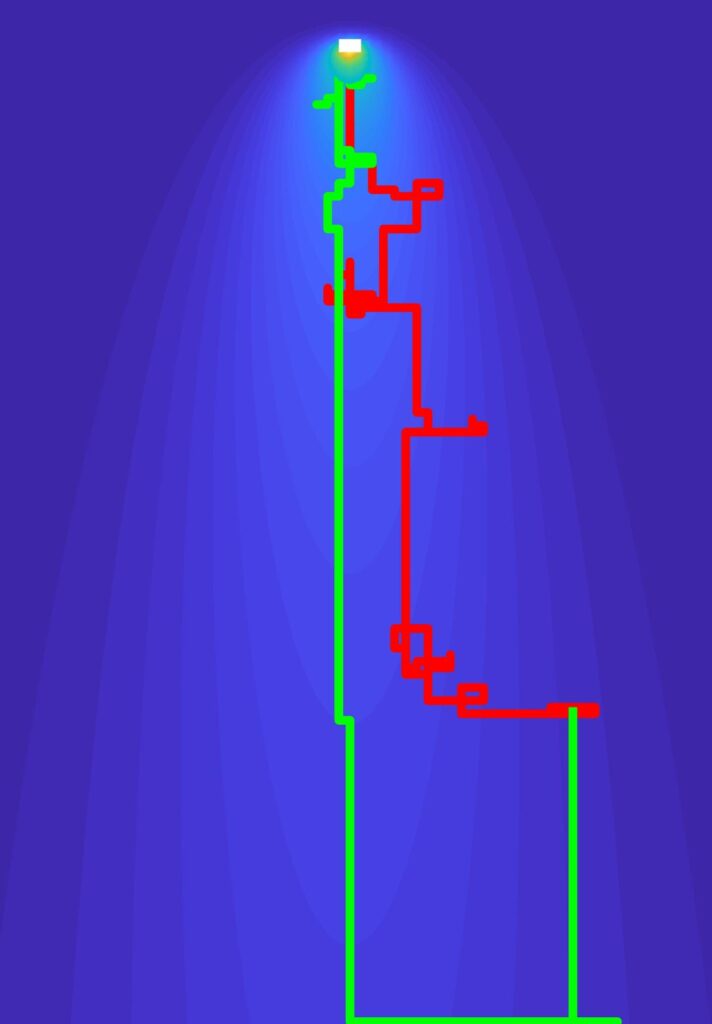
Fig. 1 Trajectories of our proposed process-aware algorithm (red) and the expected rate algorithm (green) in different scenarios. The colormap represents the mean rate of hits of the field.
- Yingke Li, Tianyi Liu, Enlu Zhou and Fumin Zhang. “Bayesian Learning Model Predictive Control for Process-aware Source Seeking”, in IEEE Control Systems Letters (L-CSS).
Risk-Aware Model Predictive Control Enabled by Bayesian Learning
The performance of a model predictive controller depends on the accuracy of the objective and prediction model of the system. Although significant efforts have been dedicated to improving the robustness of model predictive control (MPC), they typically do not take a risk-averse perspective. We propose a risk-aware MPC framework, which estimates the underlying parameter distribution using online Bayesian learning and derives a risk-aware control policy by reformulating classical MPC problems as Bayesian Risk Optimization (BRO) problems. The consistency of the Bayesian estimator and the convergence of the control policy are rigorously proved. Furthermore, we investigate the consistency requirement and propose a risk monitoring mechanism to guarantee the satisfaction of the consistency requirement. Simulation results demonstrate the effectiveness of the proposed approach.
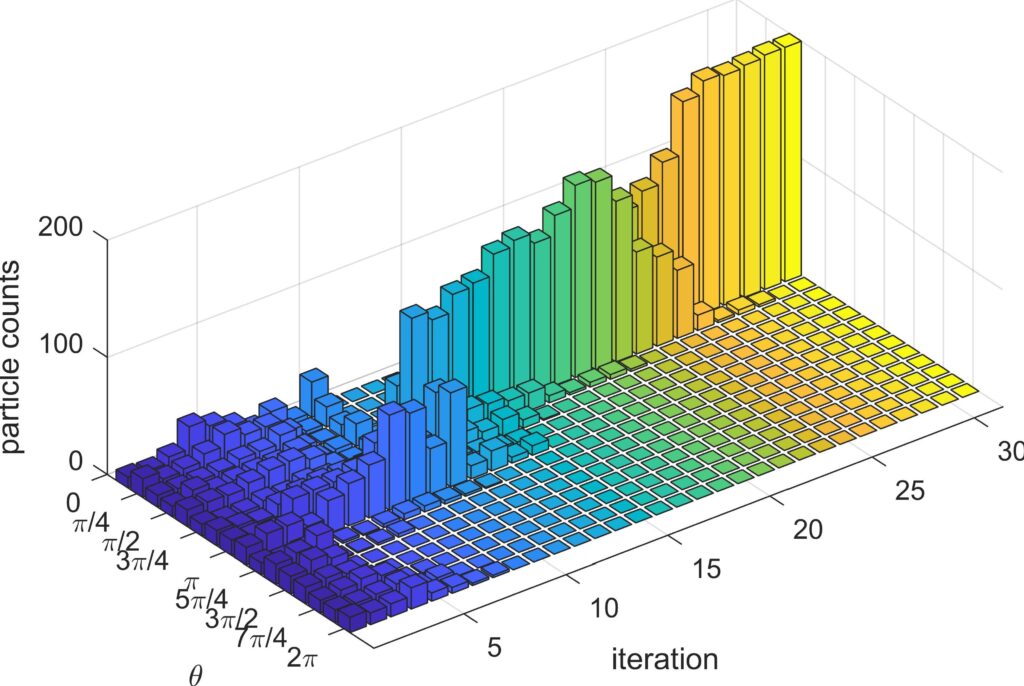
- Yingke Li, Yifan Lin, Enlu Zhou and Fumin Zhang. “Risk-Aware Model Predictive Control Enabled by Bayesian Learning”, in IEEE American Control Conference (ACC 2022).